I used to agonize about attempting to get a reasonably accurate forecast. I had all sorts of statistical models, various smoothing techniques and i even attempted to bribe/reward our sales people to provide me with accurate data to no avail.
If I over forecast and built inventory I was in the cross hairs of our accounting department for having way too much inventory and if I under-forecast sales became extremely upset that we were unable fulfill orders.
So, I typically found myself in a no-win position.
Then my ah-ha moment arrived after scheduling a meeting with a local Hospital CEO to coach them that they in essence were running a manufacturing operation and that many of the methods we used in Manufacturing could be applied within the hospital.
She quickly advised me that she knew more about Hospital Manufacturing than I did about Manufacturing.
She started with Forecasting (of course) and explained if a significant snow storm created a ton of accidents that overwhelmed emergency our manufacturing forecasting software would automatically start planning for a repeat performance 1 year out ... which of course never happened.
She also enlightened me that chasing the wrong KPI's will create a horrible business model. I will those stories for another blog post. But will tell you that out of the plethora of hospitals located in Ontario hers was the only one giving money back to the government.
However, if you are still plugging away developing your forecasts in the traditional way then the following tips will help you. But if you are focused on significantly reducing your Process Lead Time, then the need for a forecast starts to diminish along with a ton of other software tracking tools.
Richard
********************************************************************************************
1.) Using Shipment History
Sales forecasting systems use sales history data to generate the statistical forecast for future periods. The key issue is the type of sales history used to run the statistical forecast – shipment history or demand history.
Let’s say that your customer placed an order for 1,000 units of Item 12345 for delivery in July. Item 12345 is on backorder, and you are not able to ship the product until September. Does your ERP system post the sales history as July demand (when the customer wanted the product) or September demand (when you were able to deliver the product)?
If the answer is that your system posts the history as September history, your sales forecasting system will use 0 units in July and 1,000 units in September to drive the statistical forecast. Using shipment history will perpetuate your backorder situation. The appropriate procedure is to post the 1,000 units as July history for sales forecasting purposes. Avoid the First Deadly Sin and use true customer demand history to generate the sales forecast.
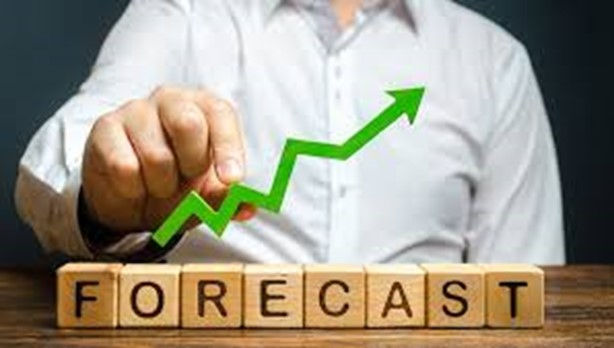
2.) Bad Data
Congratulations if you avoid The 1st Deadly Sin and use customer demand data as the basis for generating the statistical forecast. However, the demand data can still be “polluted” with the effects of one-time or non-recurring orders that can lead to inaccurate statistical sales forecasts.
Examples of the bad demand data that gets posted to the demand history file include:
• Sales due to promotions that will not be repeated in the same period next year
• Spikes in demand due to special, one-time customer orders
• Pipeline fill orders by big-box retailers
• Special orders due in advance of quarter-end price increases
• Using customer-specific demand data that is too granular to be statistically significant
• Unit of measure conversion issues, such as when item are sold as both individual units and three-packs
It is imperative that you periodically scrub the demand history file to eliminate the effect of the special orders. Some systems automatically filter demand history values that are outside of a statistical confidence interval. Other systems identify the exceptional demand and rely on the user to determine how to adjust the sales history to eliminate the bad data. The key is to include data scrubbing as part of your regular demand planning process.
3.) Excessive “Gut Feel” Overrides
Many companies commit hours, or even days, of effort each month to review and adjust the system’s statistical forecast. The sales forecast sometimes travels from the forecast planner, to the sales team, and to the product management team with each level making their adjustments to the sales forecast. Too often, planners base forecast adjustments on “gut feel” and not on specific knowledge of future customer activity. Raise a red-flag if you feel compelled to override more than 10% - 20% of the system’s statistical forecasts.
Use the system’s statistical forecast as the starting point for making forecast adjustments.
The rule of thumb should be to adjust the system’s statistical forecast only if you know something about the future that is not reflected in the demand history. Otherwise, resist the urge to adjust the forecast just to make it look pretty. Also, it is imperative to measure whether the system’s statistical forecast or the forecast adjustment is closer to the actual sales. You are likely to find that the “gut feel” forecast adjustments are actually making your sales forecast less accurate than if you had left the forecast alone.
4.) Poor Event Planning
Some companies do the opposite of The 3rd Deadly Sin of entering excessive forecast overrides. They don’t make enough forecast overrides when special events are scheduled to occur. Poor event planning is often the source of missed customer due dates, product expedites and excess inventory.
The typical special events that occur that do not have the benefit of being reflected in past sales history include:
• New item introductions
• Promotions
• Item substitutions
• Item replacements
It’s imperative to develop an internal collaboration process that brings together all of the individuals responsible for special events impact planning. You should assign clear roles and responsibilities for each individual at each step of the special events forecasting and planning process. Also, there needs to be frequent review of how actual sales are performing vs. the forecast to ensure that the right level of inventory is available to meet the special event needs.
5.) Senior Management “Meddling”
It is always interesting to see the attendee list for the weekly or monthly demand planning meeting and see the company President, Chief Financial Officer, VP Sales or VP Operations on the list. It is almost certain that The 5th Deadly Sin is sure to occur. Senior executives have no business exerting their bias or influence on the SKU forecasts.
Executive management meddling can surface in a variety of ways:
• Direct forecast overrides by executives offering their opinions during the demand planning meeting
• Threats of what will happen if service rates don’t improve (resulting in forecast increases in an attempt to increase inventory)
• Threats of what will happen if inventory is not reduced (resulting in forecast decreases in an attempt to decrease inventory)
• Forcing the budget at the product family level down to the SKU forecast as a result of the Sales &
Operations Planning process
The sales forecast should be the company’s best estimate of customer demand. It is an inappropriate technique for executives to adjust the sales forecast as a means of manipulating inventory levels and fill rates. Inventory and service levels are best managed as part of the supply planning and inventory replenishment processes.
Unfortunately, The 5th Deadly Sin of executive management meddling is often the easiest of the Deadly Sins to recognize and the hardest to do anything about.
6.) Not Measuring Sales Forecast Accuracy
It is surprising how few companies do a reasonable job of measuring forecast accuracy. When challenged to this fact, some companies respond with desperation, “even if we did measure forecast accuracy, what can we do about the large errors?” The answer is that you can do plenty about sales forecast errors. However, the process starts with measuring forecast error and understanding the root causes of high forecast errors.
Some basics of sales forecast accuracy reporting include:
• Measure forecast accuracy at the SKU and product family level
• If your product has long lead times, account for the lead time “lag” in the sales forecast accuracy reporting
• Measure which is more accurate – the system’s statistical forecast or the planners’ override forecast
• Determine if forecasts are consistently too high or too low, indicating a bias in the statistical forecast or in forecast adjustment
• Perform root cause analysis on items with high forecast errors to learn the real reasons for the forecast error
7.) Safety Stock Based on Forecast Error
Safety stock inventory exists to cover periods when actual demand is greater than the forecast. It is a common system feature to compute SKU safety stock quantities based on forecast error and a desired customer service rate. Just plug in a 98% service rate and let the system compute the safety stock quantity. Sounds too good to be true, right? Yes, until you look at the math of the traditional safety stock calculation.
The traditional safety stock calculation based on forecast error does not distinguish between periods when the forecast is too high (when we don’t need safety stock) and when the forecast is too low (when we do want safety stock).
Comments